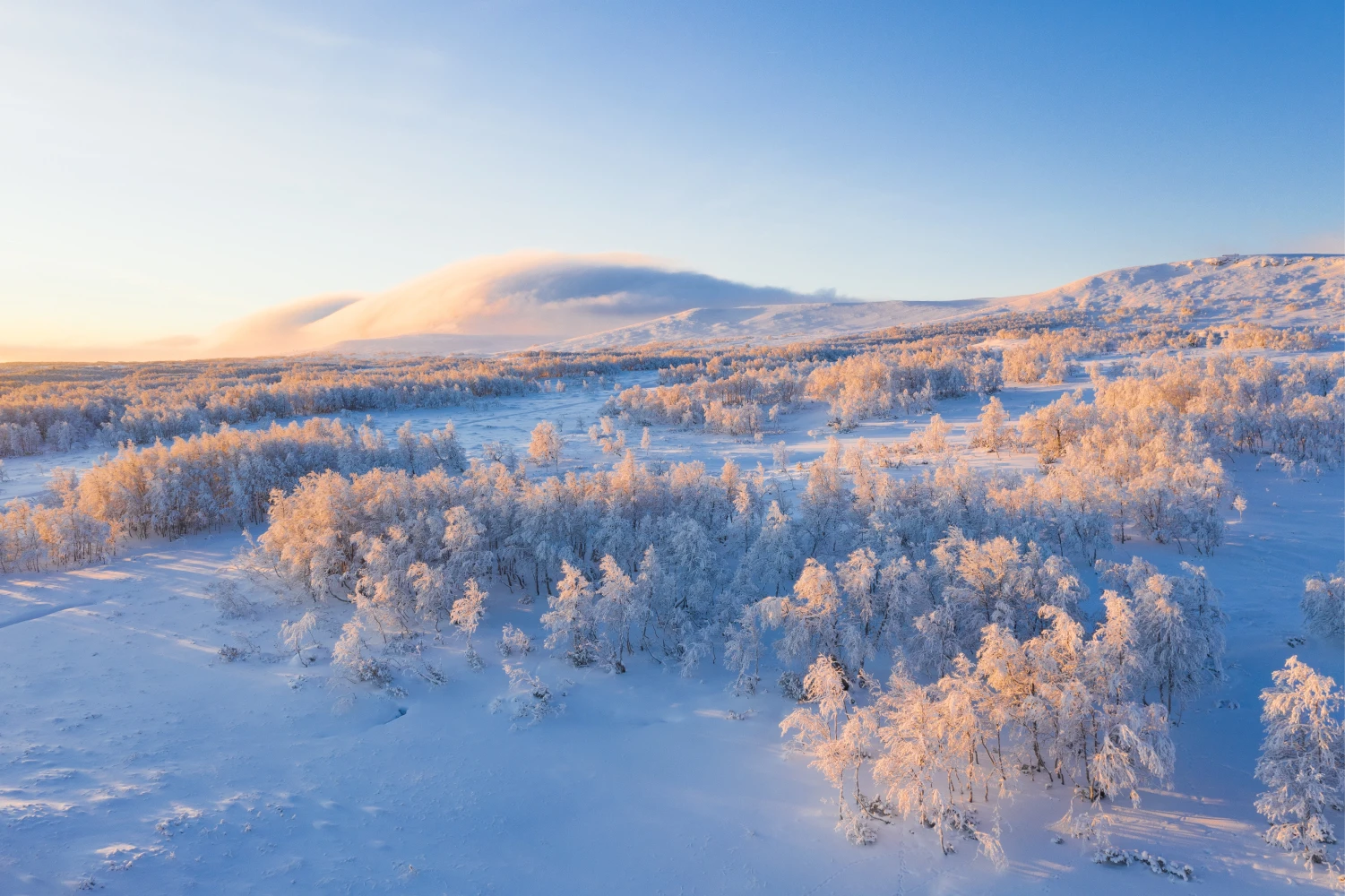
Welcome to Part 2 of our series on how to effectively leveraging AI in your business. Building on the insights from Part 1, where we introduced a 3-step methodology to identify winning AI use cases, this blog dives into the crucial next steps of prioritizing AI projects. If you haven't yet explored Part 1, we highly recommend starting there to gain a foundational understanding of how to identify winning AI opportunities.
According to a MIT study, roughly 85% of AI projects fail to deliver on expectations. A key reason? Lack of strategic prioritization.
With AI permeating business teams, the number of project ideas and possibilities can be overwhelming.
How do you thoughtfully sift through and select the AI initiatives that will provide real value and ROI?
Proper AI project prioritization is crucial for guiding your team to adopt AI successfully.
In this blog post, I'll explore a few frameworks to help you:
- Evaluate your team's AI maturity level to inform project selection
- Distinguish between quick win and big bet projects
- Conduct a structured risk-reward analysis to quantify project viability
- Apply best practices for AI project implementation
With the right prioritization approach, you can align AI initiatives to your strategic business goals, stage capabilities appropriately, and demonstrate returns to leadership.
Let's get started with assessing your organization's AI readiness. A clear view of this helps tailor project choice to your team's maturity.
Access the AI Maturity of Your Team
Assessing your team's AI maturity is akin to pinpointing your location on a journey's map, crucial for charting the course forward.
Consider a customer support team just beginning to explore AI. They could start by employing Graft's Predict App to organize customer queries, a reflection of early AI maturity.
Contrast this with an HR team well-versed in AI, perhaps utilizing Graft's advanced LLMs and AI-powered search for efficient resume screening and employee onboarding processes, indicating a more developed AI stage.
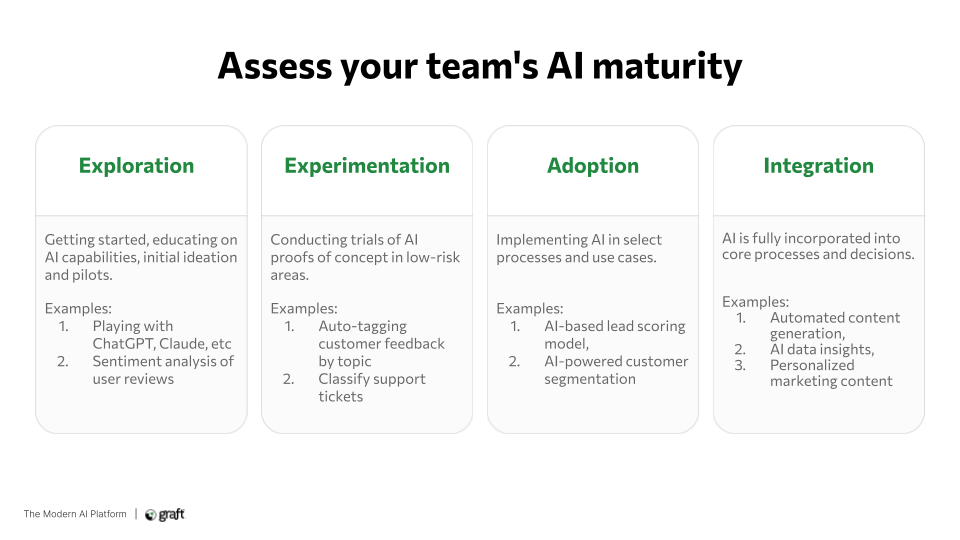
At Graft, we've seen varied examples. A product development team might be using AI for customer feedback analysis and customer segmentation, a sign of growing AI sophistication. Meanwhile, a legal team could be leveraging AI-powered search daily to find information in documents faster, showcasing deep AI integration.
Recognizing where your team stands on the AI maturity spectrum is vital. It helps in tailoring AI project choices to their current capabilities, ensuring a seamless and impactful adoption.
Graft's apps and solutions are designed to align with your team's specific AI readiness, facilitating a strategic and effective AI journey.
Categorize Your AI Projects: Quick Wins vs. Big Wins
Getting stakeholder buy-in and budget approval for AI projects can be challenging. Leaders want to see returns on investment while being cautious about overpromising.
The key is finding the right balance between 'quick wins' and 'big wins' when putting together your AI projects.
Quick wins are smaller projects that deliver rapid results while requiring minimal investment. Examples include:
- Automating sentiment analysis of user reviews
- Building a support ticket classifier to improve response times
These projects demonstrate early success and ROI for AI, helping build confidence and momentum across the organization.
Big wins are more ambitious initiatives aligned to long-term strategic objectives. While higher risk, their potential impact is transformational. Examples:
- Predictive supply chain optimization to reduce inventory costs 30%
- AI-powered personalized recommendations engine to boost sales
- Automated virtual assistant for customer service inquiries
Getting stakeholder buy-in on big wins may require thoughtful persuasion given the higher risk and resources needed. Come armed with compelling data - market projections, expected ROI based on pilots, case studies from competitors. And consider starting with a minimum viable product to validate capabilities and gain trust before going all-in.
The key is balancing quick wins to establish credibility with big bets that move the needle on business goals. This balanced portfolio gets leadership onboard while ensuring AI delivers tangible value.
With the right combination of ambitious vision and pragmatic execution, you can secure approvals and drive AI adoption for your team.
>> [FREE] How to Prioritize AI Projects Slide Deck <<
Conduct a Risk-Reward Analysis
Prioritizing AI projects is about more than just viability. It requires understanding the spectrum of risk versus reward for each initiative. This is where a structured risk-reward analysis comes in handy.
For each potential AI project, identify and quantify the major risk factors - budget overruns, resources needed, data access, regulatory barriers, etc.
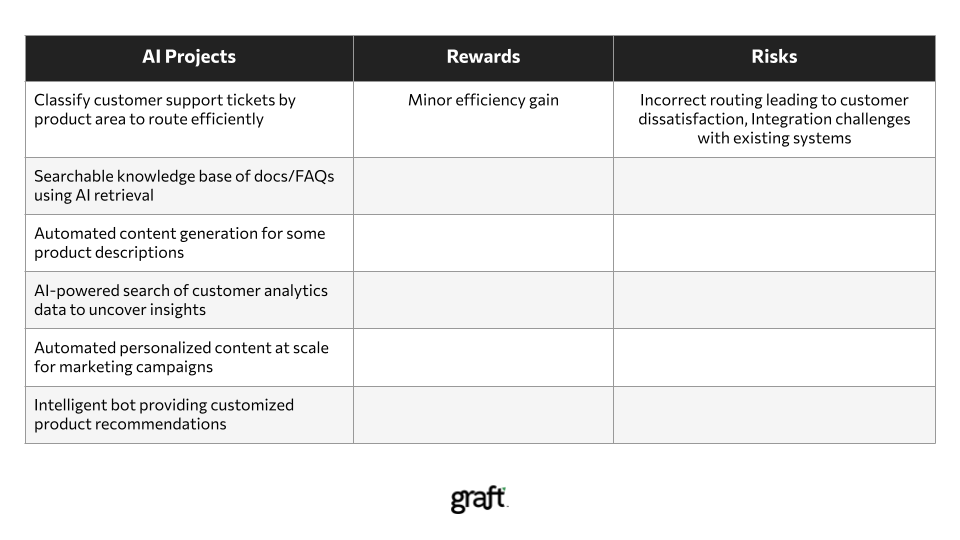
Also quantify the potential rewards - ROI, revenue increases, customer retention gains, operational efficiencies, etc. Metrics like time savings or revenue increase help quantify rewards.
The goal is to end up with a list of projects with an associated risk/reward score.
- Sentiment analysis of user reviews (Risk: 1, Reward: 3)
- Auto-tagging customer feedback by topic (Risk: 2, Reward: 3)
- Classify support tickets (Risk: 3, Reward: 4)
- AI-powered knowledge base search (Risk: 2, Reward: 8)
- AI-based lead scoring model (Risk: 4, Reward: 7)
- AI-powered customer segmentation (Risk: 3, Reward: 9)
- Automated content generation (Risk: 6, Reward: 3)
- AI data insights (Risk: 8, Reward: 10)
- Personalized marketing content (Risk: 7, Reward: 9)
- Intelligent product recommendation bot (Risk: 9, Reward: 8)
Next, plot each project on a risk-reward matrix with risk on the x-axis and reward on the y-axis.
The horizontal axis represents the risk level, ranging from low to high. The vertical axis shows the reward level, also from low to high.
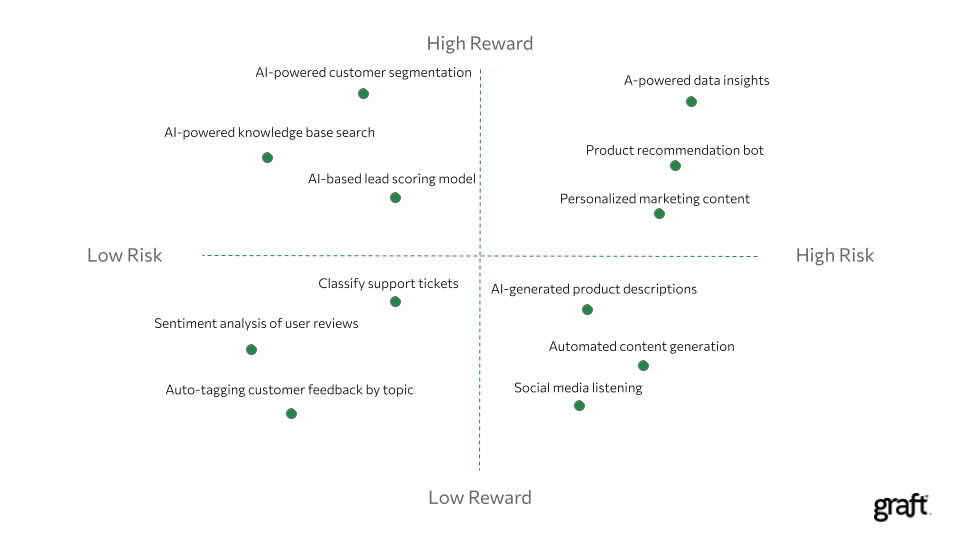
The quadrants represent different types of projects:
- Low Risk/Low Reward - "Sure Bets"
- Low Risk/High Reward - "No Brainers"
- High Risk/Low Reward - "Long Shots"
- High Risk/High Reward - "Innovative Projects"
"Quick win" tactics like searchable knowledge base of docs/FAQs using AI retrieval may fall into the "No Brainer" area, while transformative initiatives like an intelligent product recommendation bot land in the "Innovative" zone.
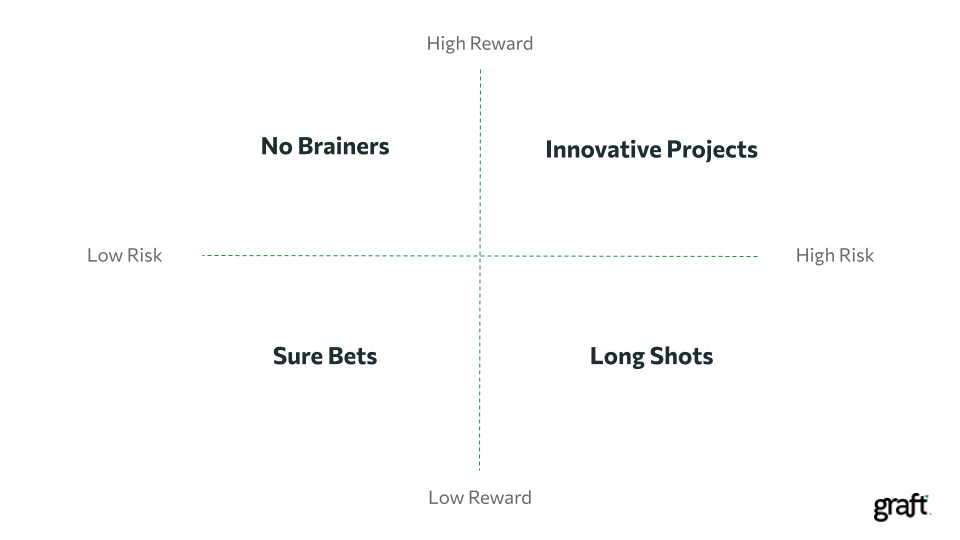
The key is to balance your AI initiatives across different risk levels, aiming for those that fall into the 'Innovative' or 'No Brainer' categories. These are the projects where the potential rewards justify the risks involved. Just remember, it's always essential to bring risks back under control and reassess as outcomes materialize.
Now it’s time to get input from diverse stakeholders to ensure you accurately capture risks and rewards from different perspectives.
By quantifying risks and rewards, plotting projects on the matrix, and managing risk, you can make smart AI project investment decisions tailored to your team's capabilities and innovation appetite, while also getting the necessary leadership buy-in to implement the project(s).
How to Implement the Risk-Reward Framework
Here are specific steps to conduct your own risk-reward analysis:
1. Identify Your AI Projects:
Start by listing all potential AI projects. This includes projects in ideation and those proposed by different teams.
2. List the Potential Risks and Rewards:
List the potential benefits (e.g., efficiency gains, revenue increase) and risks (e.g., cost overruns, technology adoption hurdles) for each AI project.
3. Quantify Risks and Rewards:
Assign a value or score to each risk and reward. Use metrics like expected ROI, impact on customer satisfaction, or time saved.
4. Map to Risk-Reward Matrix:
Plot each AI project on a risk-reward matrix based on their quantified scores to visualize where each project stands in terms of risk and potential reward.
5. Prioritize Based on Net Value:
Prioritize projects by balancing between achieving quick wins for immediate impact and investing in big wins for long-term strategic value.
6. Scenario Analysis:
Conduct 'what-if' analyses for different scenarios (e.g., best-case, worst-case) to understand how changes in risk factors impact the project's potential rewards.
7. Stakeholder Feedback:
Involve key stakeholders in the risk-reward analysis to incorporate diverse perspectives and ensure alignment with business objectives.
Following these steps ensures a structured and comprehensive approach to evaluating AI projects, helping you prioritize AI projects effectively in 2024.
Make it Happen: Key Strategies for AI Project Execution
You've assessed your team's AI maturity. You've balanced your list of AI projects between quick wins and big bets. You've plotted initiatives on the risk-reward matrix.
Now what?
With your prioritized list of AI projects, it's time to focus on execution.
Follow these best practices to drive adoption while delivering impact:
Identify Quick Wins - Look for low hanging fruit where AI can augment current processes. Examples: automating repetitive tasks, basic predictive analytics.
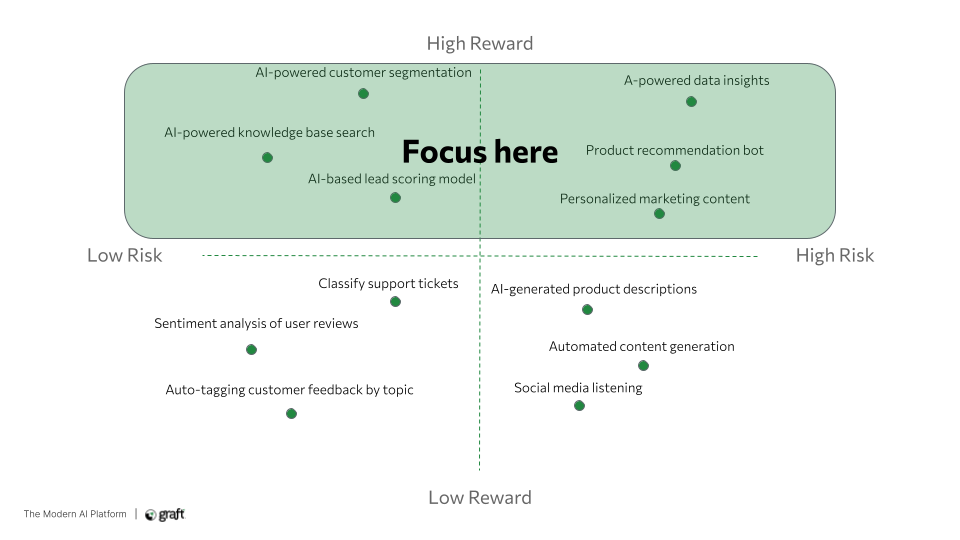
Evaluate Strategic Projects - Assess bolder AI initiatives aligned to business goals, even if higher risk. Example: AI-powered customer churn reduction.
Consider Both Risks and Rewards - Don't just focus on ROI. Also evaluate risks like implementation costs, data needs, team capability building.
Involve Cross-Functional Stakeholders - Get input from other teams like IT, operations, finance etc. to assess feasibility and alignment with broader priorities.
Start Small, Seek Quick Feedback - Pilot projects, set milestones, monitor rigorously. Pivot fast if risks increase. Don't boil the ocean early on.
Why an AI Strategy is More Than an API
Communicate and Celebrate Wins - Share positive results, learnings and AI adoption best practices across the company.
Keep Assessing as Market Evolves - Continuous evaluation essential as AI capabilities and business needs change.
Adopting these best practices ensures that your AI project prioritization is not just a one-time decision, but a continual process of strategic alignment and adjustment, resonating with Graft's ethos of fostering innovation while managing risks effectively.
Next Steps
With the right strategic approach, you can adopt AI successfully. Avoid falling into the trap of chasing "shiny new tech" without clear goals. Instead, focus on solving real business challenges with AI as an enabler.
The frameworks covered in this post will help you have those crucial discussions on aligning priorities to capabilities. You'll be able to demonstrate ROI to leadership with a balanced project portfolio.
>> [FREE] How to Prioritize AI Projects Slide Deck <<
Remember, the key to success in AI is not just in the technology itself but in how strategically and thoughtfully it is integrated into your business operations.
"At Graft, we believe that Modern AI should exclude no one. Artificial intelligence is a tide that can lift all boats, and the world will be better for it."
Adam Oliner, CEO and Founder of Graft, The Hallmarks of Modern AI
Ready to Get Started?
Now it's your turn. Gather your project ideas, assess your team's AI readiness, and start mapping initiatives on the risk-reward matrix. Develop a prioritized portfolio and roadmap to pitch to stakeholders.
By taking the first step of strategic prioritization, you'll be on your way to AI adoption success. The journey requires focus and thoughtful planning, but the rewards are immense. We're excited to be a part of your team's AI enablement.
Let's achieve AI impact together! Reach out if you need any guidance or tools for your AI project prioritization.